In-line prediction of cell cycle life performance empowers manufacturers with the ability to decrease ramp up time and increase confidence in the quality of every cell produced
The Rising Demand for Lithium-Ion Batteries and the Need for Quality Management
According to current estimates, global production capacity of Lithium-ion battery cells will increase from 1.7TWh in 2022 to 9.5TWh by 2030, primarily driven by the projected demand for EVs.1 This dramatic scale up of production capacity requires rapid ramp-up of new gigafactories with significantly lower manufacturing costs and still-unrealized levels of cell quality, reliability, and safety. For top tier battery manufacturers, data-driven quality management is a critical need to meet this demand.
Accurate in-factory prediction of battery cell performance has the power to unlock new opportunities in productivity and quality for cell manufacturers, pack manufacturers, and EV OEMs. Cell manufacturers can accelerate the development of new cell designs, rapidly validate new and efficient manufacturing processes, sort new cells by their expected lifetime, and screen out defective cells during end-of-line quality control (QC) testing. In addition, during inbound QC, pack manufacturers and EV OEMs can accelerate the validation of new cell suppliers, ensure tighter battery quality standards, and improve their ability to build higher-performance packs.
While machine learning (ML) analytics has improved manufacturing in other industries, it has not yet been widely implemented to improve productivity and quality in commercial battery factories. This is in large part due to the limited ability of standard in-process and end-of-line inspection methods to consistently and accurately predict cycle life performance of battery cells.
In this post, we discuss the ability of Liminal’s EchoStat inspection platform to use ML on spatially-resolved non-destructive ultrasound scans to accurately predict the performance of 100% of cells during production, without requiring extensive cycling.
The Need for 100% Inspection of Manufactured Battery Cells
Ensuring product quality in cell manufacturing with currently available methods poses a two-pronged challenge: First, fast tests often miss critical issues. Second, comprehensive tests are rarely fast and can only be applied to a small sample of randomly selected cells. Each leaves room for quality escapes, which can result in poor performance and field failures at best, or, at worst, safety events and vehicle recalls.
Here’s how these two challenges play out:
First: Rapid electrical QC tests like voltage, resistance, and capacity are cell-averaged scalar values that don’t tell the whole story. These methods are analogous to a doctor checking a patient’s temperature, pulse rate, and blood pressure. While these vital signs are informative, they cannot provide detailed-enough information to diagnose a specific medical condition. Similarly, rapid electrical QC tests can be used to inspect every cell, but are not sensitive to subtle internal issues that can lead to poor performance—especially as battery cells get larger.
Second: More comprehensive end-of-line testing is possible with days-long offline electrochemical tests, CT scans, and months-long cycle-life testing. However, due to their intensive nature, a random sampling of fewer than 1% of cells from each production run are typically selected for testing while the entire lot of cells is held in storage. This approach gives more detailed information compared to rapid electrical QC tests, but does not provide a complete understanding of the quality of the entire batch, leaving the door open for uncertainty and risk of defective cells slipping through without being tested. This has already led to some high-profile and expensive recalls and production halts.
As EVs become widely adopted and we demand greater performance from our vehicles, there is a need for an inspection method that delivers comprehensive information while also being fast enough to test 100% of cells produced to get a complete picture of the true distribution of batch quality and to prevent quality escapes.
The Promise of Machine Learning in Shortening Time to Information
A major bottleneck in battery manufacturing is the long time to information due to cycling. Whether it’s cell developers running designs of experiments (DOEs) to fine tune cell chemistries and internal designs, or it’s process engineers scaling up and optimizing manufacturing processes, it takes weeks to months to get feedback on the impact of parameter changes on cell performance through cycling data.
There is a big push in the battery industry to leverage ML analytics to bring a new level of insight into battery cell manufacturing to improve productivity, quality, and reliability. For example, researchers showed that ML models can use data from 50 to 100 cycles of charge-discharge testing to accurately predict longer-term cell performance. However, this still takes several days and as cycler channels are a limited resource in battery cell factories, cycle-testing 100% of manufactured cells is simply not feasible.
In short, while ML has shown promise for reducing time to information, better input data is required to unlock its true potential for impact in high-volume battery cell manufacturing.
Introducing EchoStat: Innovation in Battery Inspection and Cycle Life Prediction
Liminal’s EchoStat solution is uniquely able to provide information on expected long-term performance for 100% of cells produced in real-time during production, rather than waiting for weeks to months of cycling. This allows for faster production ramp up with more efficient test-and-validation plans and increased confidence in production quality and reliability.
EchoStat ARRAY is our proprietary high-speed system for in-line cell inspection. Currently, each measurement takes six seconds per battery cell, is compatible with any flat cell format (pouch and prismatic), works with any cell chemistry (e.g. LIB, Li-metal, Na+ ion, etc.), is fully automated, and can be integrated into a production line to inspect 100% of manufactured cells.
The illustration below shows how this works. A well-defined ultrasound signal is transmitted into one side of the cell. As it travels across the cell and interacts with all the internal materials, the ultrasonic wave picks up information about the cell’s physical properties. The resulting signal is received by a sensor on the other side of the cell.
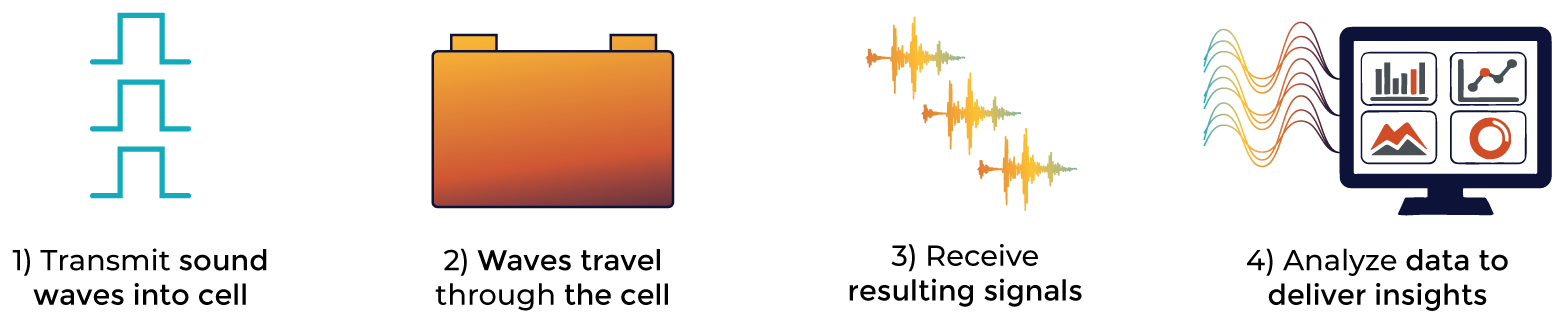
Spatially resolved measurements are achieved by using multiple sensor pairs placed at positions across the entire face of the cells. This approach allows us to capture data on internal inhomogeneities in the battery cells, which are often the cause of poor performance and early failures. We then transform this rich dataset into actionable insights using our edge ML analytics.
One example of our edge analytics is our Cycle Life Prediction (CLP) model, which leverages EchoStat data collected during production to predict how a cell will perform, without putting it through any charge discharge testing. The results of Liminal’s CLP solution versus electrical QC-based prediction on a batch of commercial Li-ion battery cells are shown in the figure below.
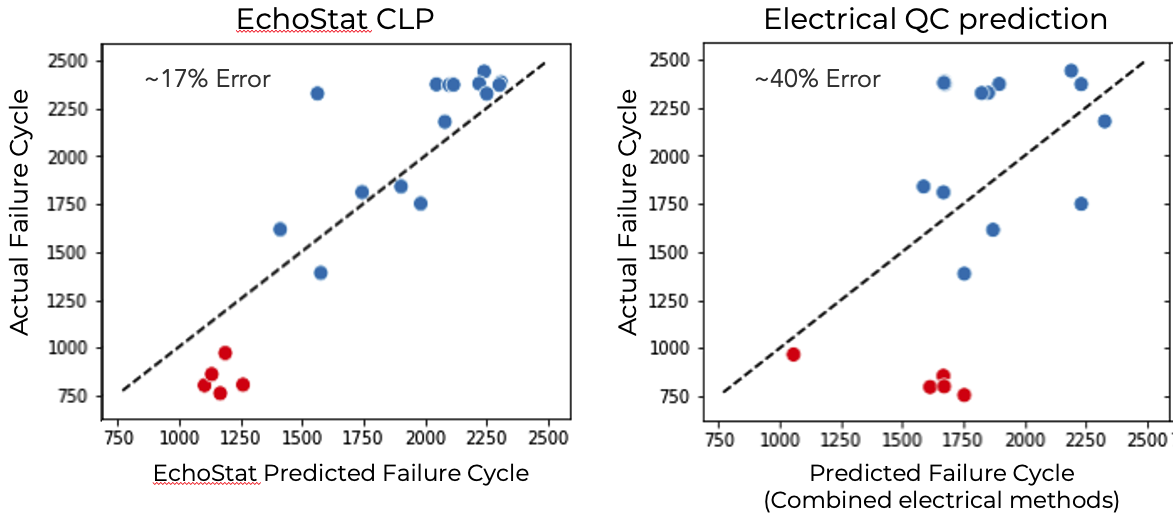
This figure depicts the actual failure cycle2 number during extended charge-discharge testing versus the failure cycle number predicted by EchoStat CLP using ultrasound data collected after formation (left) and by a combination of standard electrical QC metrics including formation capacity, DCR, ACR, and OCV drop during aging (right). If the prediction were 100% accurate, all the dots would lie on the 45-degree dashed line. The blue dots depict cells that followed a gradual capacity fade curve, while the red dots represent cells that experienced a rapid drop in capacity, likely due to manufacturing defects or issues present in the cells.
This data shows that EchoStat CLP predicted the failure cycles of these Li-ion pouch cells with greater than 80% accuracy or more than 17% mean absolute percent (MAP) error. In contrast, the electrical QC predicted the failure cycles with only ~60% accuracy or ~40% MAP error and were additionally not able to discern which cells would experience sudden failure.
We have also demonstrated that this method works on various commercial cell types including Li-ion pouch cells, Li-metal pouch cells, and large Li-ion prismatic cells. For each, we’ve shown that EchoStat CLP can accurately predict cells’ cycle life performance in just a few seconds per scan, enabling 100% in-line or end-of-line inspection during production. To learn more about EchoStat cycle life prediction, stay tuned for our upcoming white paper on the topic.
From Ultrasound to Insights: Leveraging Physics-Assisted ML for Cycle Life Prediction
EchoStat data processing and analysis is not a “black-box” solution that relies solely on unsupervised learning and big data. Instead, we take a physics-assisted ML approach to model training. This allows us to build more robust models and also to understand the “why” behind our predictions.
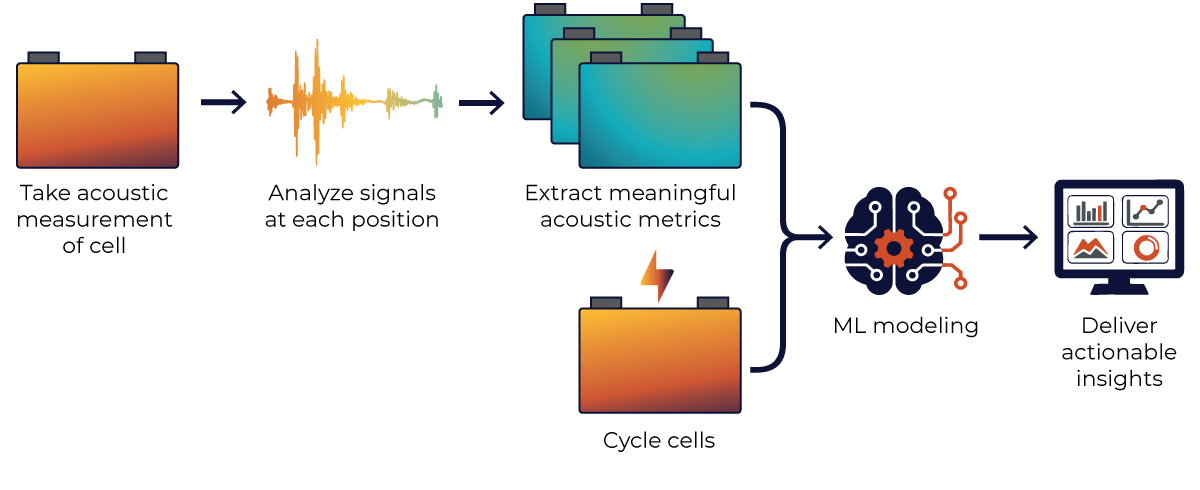
To achieve this, we first extract high-dimensional acoustic metrics from the ultrasound signal collected at each position across the cell area. Then, for model development, we carefully select the most relevant acoustic metrics based on a deep understanding of sound physics and the underlying physics of the cells. The cells in the training set are cycled to produce a ground truth, which is used to generate the model that—once applied—can predict cell cycle life with a fast ultrasound snapshot, e.g. after formation or at the end-of-line. This approach has enabled us to achieve high accuracy in our predictions and deliver robust, actionable insights during cell production.
EchoStat Cycle Life Prediction Unlocks Opportunities Across All Stages of Battery Manufacturing
The ability to accurately predict cycle life performance has major benefits at every stage of battery cell production. For cell developers, fast feedback on cell performance can dramatically reduce the amount of time spent on bottleneck processes and accelerate iterations in DOEs. For process engineers, rapid indication of cell performance accelerates production ramp up, enables faster yield improvements, and expedites root cause analysis of process issues. Finally, in series production, the ability to predict lifetime performance with 100% cell inspection provides a comprehensive understanding of production stability, reduces costly escapes, and increases confidence in product quality and reliability. All of this results in huge cost savings and serves to help the industry as a whole rapidly scale up new battery factories with advanced quality management to meet the accelerating demand for EVs world wide.
To learn more, visit our contact page and subscribe to our newsletter.
Sources and Definitions
1benchmarkminerals.com/market-assessments/gigafactory-assessment
2Here, “Failure Cycle” is defined as the number of cycles to hit 80% of initial capacity retention or the cycle number when the cell shorts.