Scaling production is an essential part of growth in any industry, but it’s not as simple as just adding new equipment and processes to your production line. Floorspace, infrastructure, workforce, and supply chain all have to scale too. The act of increasing production inherently entails a comprehensive increase in variability, bringing more potential pitfalls to the surface. In this post, we’ll talk about some of the concerns around scaling EV battery manufacturing and how manufacturers can address soaring demand in the near term.
Scale Production, Not Problems
Scaling production is like baking. When you bake a cake, you’ll probably add some flour, eggs, milk, butter, sugar and salt. Maybe some other ingredients too. But you can’t just throw them all in a bowl and set the mixer to high. Baking is a science; each ingredient needs to be added in just the right way to achieve the desired result.
Naturally, your production line is similar in that every step must be executed precisely and consistently. Now imagine you multiply that cake recipe by two or three times. You can’t simply multiply the ingredients. You meticulously adjust the recipe to account for new variables that emerge from scaling. Ingredients may not interact the same way at larger volumes, necessitating tweaks to ensure the final product is as delicious as the original. Again, scaling manufacturing is similar—it’s not just a question of how much you’re producing, but also the processes that you put in place. Scaling production brings an exponential increase in issues and difficulties rather than a linear one. To scale effectively, it’s important to think of your manufacturing line as a product, rather than simply a process.
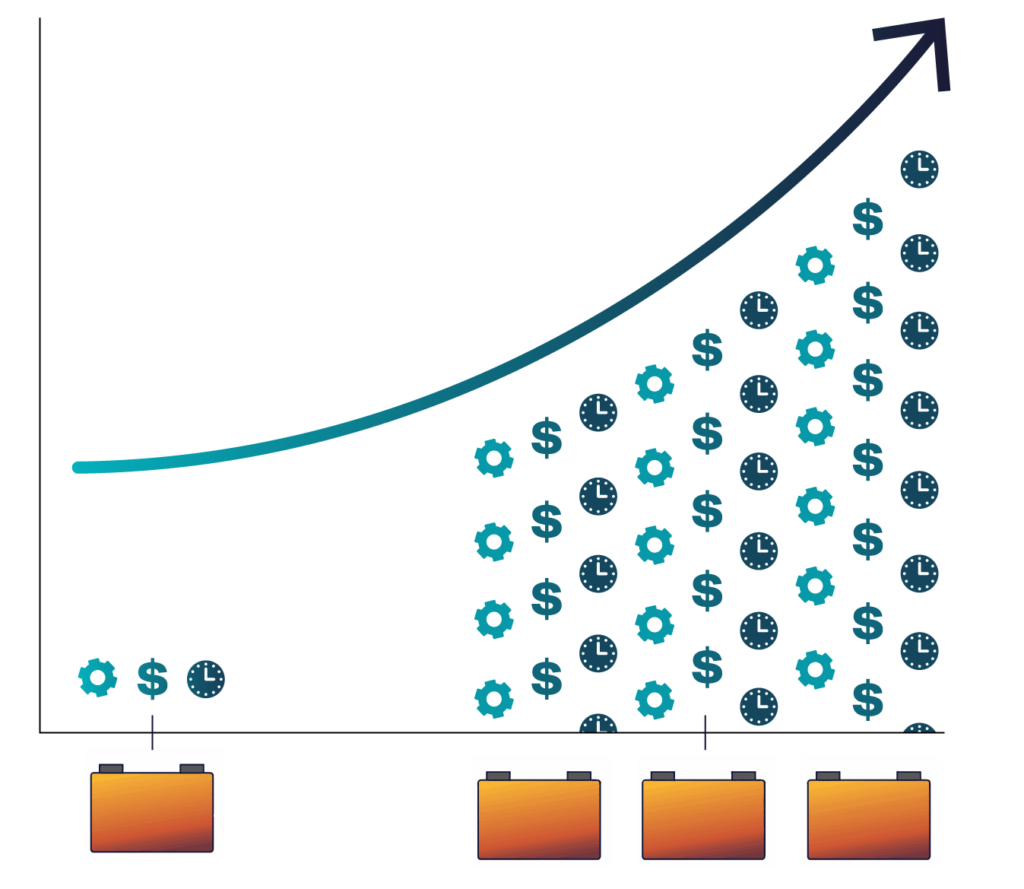
Manufacturers are hard at work trying to address the exponential challenges of scaling production, but it remains an incredibly complex web of problems to solve. A primary reason for this is that legacy inspection solutions don’t provide plant managers with sufficient information to address all of these issues in a timely manner. Lack of real time data, problems with focusing on end-of-line QA, and ramp up issues due to the scarcity of actionable information are all major roadblocks to successfully scaling production.
Current Cell Quality Testing Methods Don’t Tell the Whole Story
Current testing methods are time-intensive, yet they’re unable to capture a complete picture of cell health or test 100% of the cells being produced. As we discussed in a previous post, rapid electrical QC tests like voltage, resistance, and capacity are cell-averaged scalar values. This standard approach, while rapid, often lacks the sensitivity to detect important internal issues that can lead to poor performance or potential safety risks.
More comprehensive end-of-line testing requires days-long offline electrochemical tests, CT scans, and months-long cycle-life testing. In this instance, random sampling of typically fewer than 1% of cells from each production run are selected for testing while the entire lot of cells is held in storage. This approach provides a greater depth of information, but not the breadth to fully understand the quality of the complete batch. These inefficient testing methods can have a negative impact on the entire manufacturing process, resulting in large amounts of waste, long production timelines and bottlenecks along the way, and occasionally defective cells slipping through without being tested, which can lead to dangerous malfunctions or costly recalls that further hamper the process. As EV battery manufacturers look to scale up production, these issues threaten to become exponentially more severe. This highlights the urgent need for a comprehensive inspection solution, combining speed, precision, and coverage, to give a fuller picture of cell health.
Reimagine Your Production Line With EchoStat®
New manufacturing plants are constantly being built and scheduled for construction around the world. In fact, a recent report from the U.S. Department of Energy in conjunction with Argonne National Laboratory projects the wave of new planned EV battery plants will increase the battery manufacturing capacity of North America from 55 GWh in 2021 to nearly 1,000 GWh/year by 2030. In Europe, total production capacity of lithium-ion batteries was projected to reach 1,800 GWh by 2030—though Transport & Environment has warned that number may drop by more than two-thirds, due to delays or cancellations in new plants coming online. As these statistics show, it can take several years for new plants to be completed (if at all), let alone reach their maximum production potential. As EV battery demand continues to grow and manufacturers look for ways to meet demand in the near-term, there is an increasingly pressing need for innovative solutions.
Our EchoStat® platform integrates ultrasound technology and physics-based machine learning in a first-of-its kind battery cell testing solution. EchoStat provides real-time data insights, facilitates process optimization, and equips manufacturers with the tools to scale up production without compromising quality or efficiency. To revisit our baking analogy: If the production line is an intricate cake recipe, EchoStat acts as the master chef, overseeing every step of the process. It knows exactly what the mix should look like before, during and after baking—identifying the tiniest inconsistencies at each stage and giving direction on how to make adjustments in real-time for the best possible outcome. During battery cell production, EchoStat keeps a vigilant eye and can identify potential anomalies with greater than 80% accuracy. In end-of-line quality control testing, EchoStat is 95% faster than electrochemical tests and can achieve 100% inspection coverage, ensuring your process runs smoothly and produces consistent results as you scale, improving production efficiency, reducing cost and eliminating wasteful errors along the way.
When it comes to scaling your production, don’t scale your problems too. Integrate our first-of-its-kind, automated, non-destructive inspection solution. Reimagine your production line with EchoStat.